- What is data and analytics for businesss?
- Importance of Data and Analytics for business
- How does Data Science and Analytics Work?
- Industrial Applications of Data Science
- Challenges that businesses face while implementing Data Science and Analytics
- Tools that can help implementation of Data Science & Analytics for businesses
- Future of Data Science and Analytics
- How Ways and Means Technology can help you implement Data Science and Analytics in your business ?
What is data and analytics for businesss?
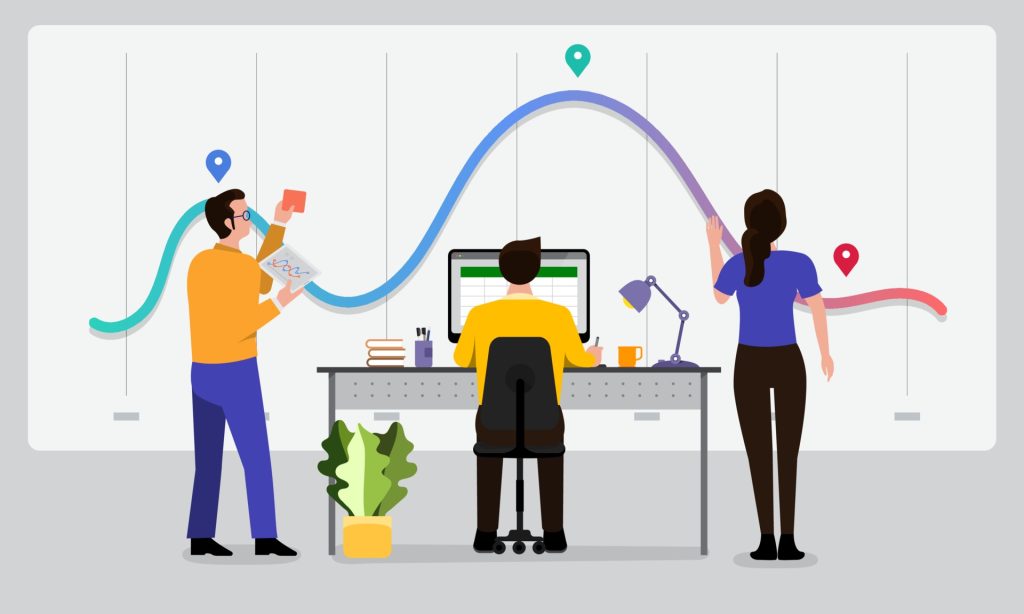
The use of data science methodologies and analytical tools to glean useful insights and arrive at data-driven decisions in a business environment is referred to as “data science and analytics for businesses.” Large volumes of data must be gathered, examined, interpreted, and visualised in order to find patterns, trends, and correlations that might enhance corporate growth and decision-making.
Predictive modelling, machine learning, statistics, and other fields are all included in the field of data science. Businesses may make sense of complicated and varied data sources, including customer information, sales data, social media interactions, website traffic, and more, by utilising these approaches. As a result, they are better able to comprehend their clients, market trends, operational effectiveness, and other important facets of their organisation.
Analytics is essential to data science for businesses because it offers tools and processes for efficient data processing and analysis. Through the use of statistical analysis, data visualisation, and numerous modelling techniques, it entails turning raw data into insightful discoveries. These insights support businesses in a variety of ways, from strategic planning to day-to-day operations, by assisting them in identifying opportunities, streamlining processes, reducing risks, and improving decision-making.
There are various advantages to incorporating data science and analytics into corporate operations. It enables companies to:
- Make educated judgements: Rather than depending entirely on instinct or gut feeling, data-driven insights allow organisations to make educated decisions that are supported by data and statistical rigour.
- Discover patterns and trends: By analysing massive databases, firms can discover correlations, patterns, and trends that might not be immediately obvious. Understanding consumer behaviour, market dynamics, and new business opportunities are all aided by this.
- Increase operational effectiveness: Organisations can optimise operations and cut costs by using data science and analytics to find bottlenecks, inefficiencies, and opportunities for improvement in business processes.
- Improve customer experience: By studying customer data, organisations can learn more about the preferences, actions, and requirements of their clients. This data can be utilised to tailor marketing campaigns, increase product selections, and improve customer service in general.
- Reduce risks and fraud: Data science methods may assist in real-time risk and fraud detection, allowing companies to put preventative measures and security processes in place to protect their operations and assets.
- Promote innovation: By seeing fresh opportunities, creative ideas, and potential market gaps, data science and analytics help organisations stay one step ahead of the competition and promote innovation within their sector.
In conclusion, data science and analytics have evolved into crucial business tools that help organisations capture the power of data and turn it into insights that can be used to boost productivity, promote innovation, and drive growth.
Importance of Data and Analytics for business
For a number of reasons, data and analytics are essential for businesses.
- Making educated judgements: Data and analytics give firms insightful data and fact-based knowledge, enabling them to make decisions. Businesses can utilise data to understand client preferences, market trends, and operational performance rather than relying on intuition or guesswork. This enables them to make strategic and tactical decisions that are more likely to result in success.
- Competitive advantage: Companies that can successfully use data and analytics have an advantage in today’s data-driven environment. Businesses can find patterns, trends, and opportunities by analysing vast amounts of data that may not be obvious to their rivals. This enables them to keep on top of trends, foresee market developments, and promptly alter their plans, goods, and services.
- Greater operational effectiveness: Analyses of data and information can reveal inefficiencies and procedural bottlenecks in business operations. Businesses may find areas for improvement, streamline processes, and optimise workflows through data analysis, which boosts output, lowers costs, and better allocates resources.
- Deeper customer understanding: Data and analytics help companies comprehend their clients better. Businesses can determine client preferences, purchasing patterns, and needs by analysing customer data. By customising product offerings, marketing efforts, and customer service, this data can enhance consumer satisfaction. Businesses may strengthen client relationships and increase consumer loyalty by offering more relevant and customised experiences.
- Identifying and mitigating risks and detecting fraud are made possible by data and analytics. Businesses can discover possible risks like operational inefficiencies, supply chain interruptions, or cybersecurity concerns by analysing historical and current data. Analytics can be used to identify patterns and anomalies linked to fraudulent activity, allowing firms to take preventative action and lessen the effects of fraud.
- Innovation and new opportunities: Data and analytics may help firms identify new prospects and foster innovation. Businesses can find market gaps, uncover unmet customer demands, and create creative goods or services to fill them by analysing market data, customer feedback, and developing trends. New business models, alliances, and revenue streams can all be sparked by data-driven insights.
- Accurately measuring and tracking performance: Data and analytics give businesses the tools they need to do just that. Establishing key performance indicators (KPIs) and using data to monitor business goal progress, assess the success of strategies and initiatives, and pinpoint areas that need improvement or optimisation are all possible.
Overall, in the digital age, data and analytics are crucial for enterprises. Better decisions may be made, a competitive advantage can be gained, operational efficiency is increased, customer experiences are improved, risks are reduced, innovation is stimulated, and performance is measured. Businesses may generate priceless insights that fuel growth, profitability, and long-term success by utilising data properly.
How does Data Science and Analytics Work?
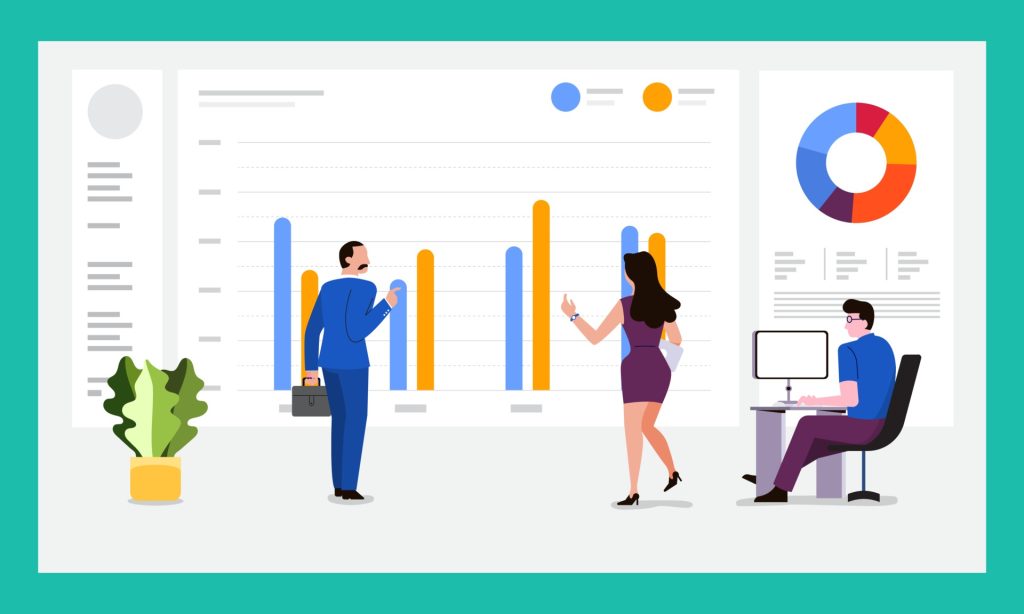
Data science and analytics use a methodical approach that entails a number of stages to draw conclusions and create value from data. An overview of data science and analytics at a high level is provided below:
- Problem formulation OR Asking the right questions: The initial stage is to specify the business issue or goal that data analysis is intended to solve. Understanding the objectives, finding the most important questions, and figuring out how data may help with problem-solving are all necessary steps in this process.
- Data gathering OR Gathering relevant data: After the issue has been identified, pertinent information must be gathered. Data can come from a variety of sources, including sensors, social media platforms, databases, spreadsheets, and other organised and unstructured sources. During the gathering phase, it’s crucial to guarantee data quality, accuracy, and proper data governance procedures.
- Data pretreatment OR Cleaning and transforming the data: To make raw data acceptable for analysis, preprocessing is frequently necessary. Data cleaning is the process of addressing missing values, fixing mistakes, and handling outliers. To maintain consistency and compatibility across various data sources, data may also need to be processed, standardised, or aggregated.
- Exploratory data analysis (EDA) OR Understanding the data through visualization and summary statistics: EDA is the process of evaluating data in order to get preliminary insights, comprehend its features, and spot patterns or linkages. To identify important features, distributions, correlations, and outliers within the data, EDA techniques include summary statistics, data visualisation, and data profiling.
- Feature engineering OR Selecting and creating meaningful features : From the data that is provided, useful features (variables) are chosen, modified, and created. The goal of this approach is to gather the most pertinent data possible to help create reliable and precise predictive models. To decide which attributes are most informative for the specific problem, domain expertise is frequently needed.
- Model development OR Applying data science techniques and algorithms: Predictive or descriptive models are developed in this step using a variety of data science techniques. These methods may use advanced artificial intelligence techniques, machine learning algorithms, or statistical models. The type of the problem, the data at hand, and the specific business goals all influence the model selection.
- Validation and training of models OR Optimizing and assessing model performance: Models are validated using sets of data that are often divided into training and validation sets. Model parameters are optimised during training to better suit the data and reduce mistakes. The model’s effectiveness, generalizability, and hyperparameter adjustments are tested, evaluated, and improved upon using the validation set.
- Model evaluation OR Measuring the effectiveness of the models: Depending on the particular issue, different evaluation metrics (such as accuracy, precision, recall, or mean squared error) may be used to assess a model. To analyse a model’s efficacy and applicability, its performance is compared to the specified business objectives.
- Model deployment and implementation OR Putting the models into action: Once a model is judged to be adequate, it is put into a production setting where it can be used to predict the future, uncover new information, or assist in making decisions. The model may need to be integrated into already-existing systems, user interfaces may need to be developed, and automated procedures for data updates and model retraining may need to be developed.
- Monitoring and maintenance OR Tracking model performance and making necessary updates: It’s important to keep an eye on deployed models to make sure they keep working well over time. This entails monitoring their efficacy, spotting performance deterioration, and upgrading models when fresh data becomes available or as the business context changes. In order to maintain the models’ applicability and dependability, ongoing maintenance is essential.
Data scientists and analysts use a variety of statistical approaches, machine learning algorithms, programming languages (such Python or R), data visualisation tools, and domain expertise throughout the entire process to extract insights, construct models, and effectively communicate findings.
It’s crucial to keep in mind that the precise workflow and tactics employed can change depending on the particular challenge, the data at hand, and the business context. As new insights or issues surface, data science and analytics are iterative processes that frequently include modifying and going over previous procedures.
Industrial Applications of Data Science
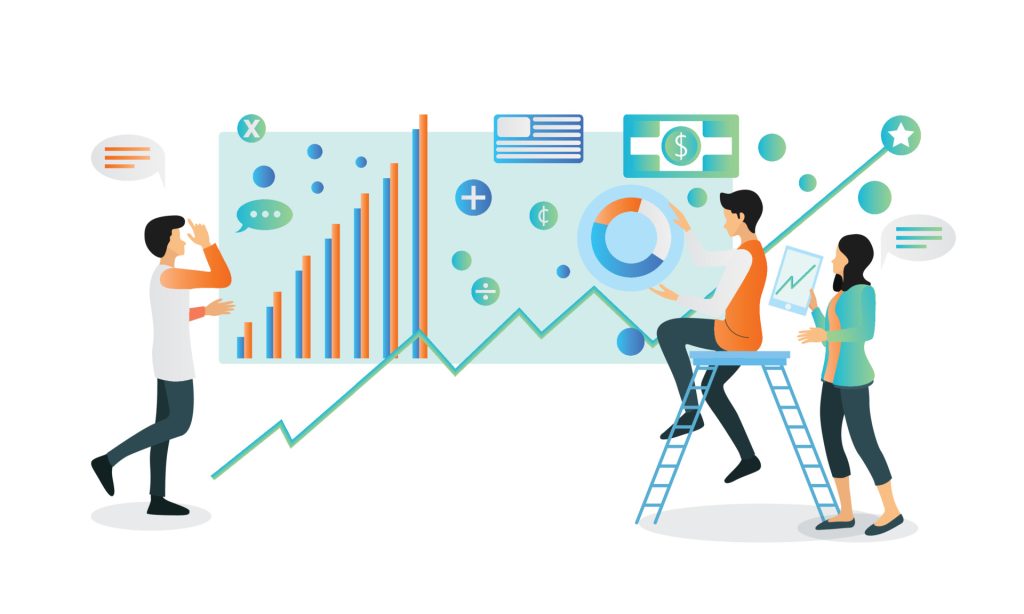
- Retail and E-commerce:
- Customer segmentation and personalized marketing
- Demand forecasting and inventory optimization
- Recommender systems for product recommendations
- Fraud detection and prevention
- Price optimization and dynamic pricing
- Finance and Banking:
- Credit scoring and risk assessment
- Fraud detection and anti-money laundering (AML)
- Customer churn prediction and retention strategies
- Portfolio optimization and investment strategies
- Fraud detection and prevention
- Healthcare and Pharmaceuticals:
- Predictive analytics for disease diagnosis and treatment
- Patient monitoring and personalized medicine
- Drug discovery and development
- Health insurance fraud detection
- Supply chain optimization for pharmaceutical distribution
- Manufacturing and Supply Chain:
- Predictive maintenance for machinery and equipment
- Quality control and defect detection
- Supply chain optimization and demand forecasting
- Inventory management and optimization
- Process optimization for improved efficiency
- Energy and Utilities:
- Predictive maintenance for power grids and equipment
- Energy demand forecasting and load balancing
- Asset management and optimization
- Renewable energy optimization
- Fraud detection and energy theft prevention
- Telecommunications:
- Customer churn prediction and retention strategies
- Network optimization and capacity planning
- Customer sentiment analysis and social media analytics
- Fraud detection and prevention
- Targeted marketing and personalized offers
- Transportation and Logistics:
- Route optimization and fleet management
- Predictive maintenance for vehicles and equipment
- Demand forecasting and supply chain optimization
- Real-time tracking and logistics optimization
- Fraud detection and prevention
- Marketing and Advertising:
- Customer segmentation and targeting
- Campaign optimization and A/B testing
- Sentiment analysis and social media analytics
- Customer lifetime value prediction
- Marketing attribution and ROI analysis
- Insurance:
- Risk assessment and underwriting
- Fraud detection and claims analysis
- Customer segmentation and personalized offerings
- Pricing optimization and premium modeling
- Loss reserving and actuarial analysis
- Government and Public Sector:
- Fraud detection and prevention in benefit programs
- Predictive analytics for crime prevention and public safety
- Traffic optimization and smart city initiatives
- Resource allocation and demand forecasting
- Social welfare program optimization
These are but a handful of the countless industrial uses for data science and analytics. Data requirements for various organisations and sectors vary, and data science methodologies can be customised to meet particular problems and possibilities in each industry.
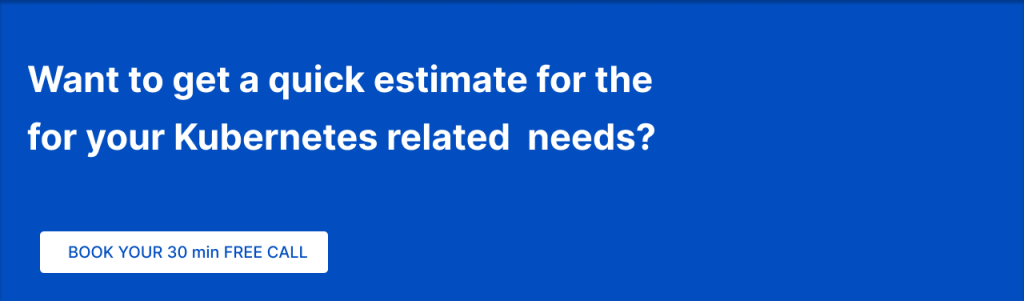
Challenges that businesses face while implementing Data Science and Analytics
For firms, implementing data science can bring a number of difficulties. Here are some typical difficulties:
- Data quality and accessibility: Ensuring the availability of high-quality data is one of the main problems. Data that is inaccurate or inconsistent can make it difficult to do thorough analysis and modelling. Additionally, it might be difficult and time-consuming to collect pertinent data from numerous sources and combine it into a single format.
- Ensuring data privacy and security is essential since organisations manage significant amounts of sensitive data. In order to comply with data protection laws like the GDPR or CCPA, strict security controls and data management that upholds moral standards are necessary. It might be difficult to put effective data governance practises into place and to build secure infrastructure.
- Talent and knowledge: Data science calls for specialised knowledge and abilities. Given the growing demand for data scientists, analysts, and engineers, it can be difficult to find and keep these people. Although challenging, developing a data-driven culture within the organisation and assembling a capable data science team are essential.
- Technology and infrastructure: Data science implementation frequently requires a strong technological infrastructure. Powerful hardware, expandable storage, and effective data processing systems are all examples of this. The right technologies, such as big data frameworks, analytics tools, and cloud computing, must be purchased by organisations, and their integration with current systems must be guaranteed.
- Change management and organisational culture: Adopting data-driven decision-making may necessitate substantial changes in organisational procedures and culture. Challenges can include resistance to change, a lack of data literacy among staff, and poor teamwork and communication. A culture that values data-driven insights and encourages the incorporation of data science into decision-making processes must be promoted.
- Return on investment (ROI): Putting data science ambitions into practise may need large investments in terms of infrastructure, personnel, and resources. It can be difficult to show a profitable return on investment, particularly early on when real results might not be apparent for some time. This problem can be solved by connecting data science activities with distinct business goals and developing quantifiable success measures.
- Scalability and complexity: Scalability becomes an issue when firms expand and produce more data. It is crucial to make sure that data science procedures and models can handle rising data quantities and complexity. Long-term success depends on creating scalable programmes and frameworks that can manage massive amounts of data processing and analysis.
- Ethical considerations: Data science poses moral questions about fairness, accountability, and bias. Data biases can provide biassed results and decisions, which might have negative implications. Businesses always face the difficulty of ensuring justice and accountability in data science models and overcoming potential biases.
It takes a systematic approach, sufficient funding, and dedication to data-driven decision-making to overcome these obstacles. In addition to developing a culture that appreciates and supports data science, organisations need to make investments in data governance, talent development, and technology infrastructure. To effectively handle issues and get the most out of data science programmes, collaboration between data science teams, subject matter experts, and business stakeholders is essential.
Tools that can help implementation of Data Science & Analytics for businesses
Enterprise data science has a variety of tools at its disposal that can be used at various phases of the data science workflow. Listed below are some typical tools:
Apache Kafka is a distributed streaming framework for managing high-volume, real-time data streams.
- Data Integration and Management.
- Apache NiFi: A user-friendly tool for data integration that allows for the gathering, transforming, and routing of data between systems.
- Talend: A complete data management and integration platform with several interfaces and data processing options.
2. Exploration and Visualisation of Data:
- Tableau: A well-liked application for data visualisation that enables users to build interactive dashboards and visualisations using a variety of data sources.
- Data exploration, interactive reporting, and visual analytics are all made possible by the business intelligence platform Power BI from Microsoft.
- QlikView and Qlik Sense: Tools for data exploration and visualisation that offer simple and interactive visualisations.
3. Model building and Experimentation
- Python is a sophisticated programming language with robust libraries like Pandas, NumPy, and Scikit-learn that is frequently used for data analysis, machine learning, and model building.
- R: A programming language used frequently for data analysis and modelling that was created primarily for statistical computation and graphics.
- Popular deep learning frameworks TensorFlow and PyTorch offer a wide range of capabilities for creating and refining neural networks.
4. Collaborative Workspaces and Version Control:
- Git is a popular version control tool that enables group collaboration on the creation and administration of code and models.
- GitHub and GitLab are web-based platforms that use Git for project management, collaboration, and version control.
- Data, code, and models may all be versioned and reproduced using the DVC (Data Version Control) version control system, which was created expressly for data science projects.
5. Monitoring and Model Deployment:
- Docker: A technology for containerization that makes it possible to package models and the dependencies they require for quick deployment and reproducibility.
- Kubernetes: An open-source system for managing and scaling the deployment of containerized applications.
- MLflow: An open-source tool for managing the entire machine learning lifecycle, including model tracking, testing, and deployment.
These are just a few examples of the enterprise data science tools that are accessible. The organization’s particular demands, tastes, and technical requirements all play a role in the tool selection. Organisations frequently combine these technologies to build a thorough data science ecosystem that supports their particular data analytics and modelling needs.
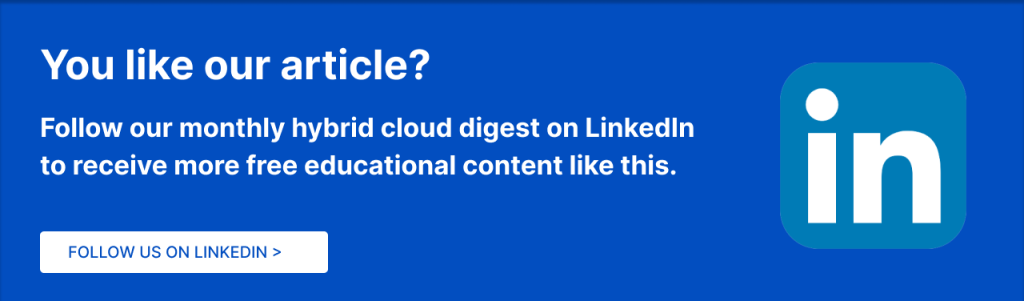
Future of Data Science and Analytics
Data science has a bright future and has a great deal of room to grow and innovate. The following significant factors illustrate the upcoming developments and prospects in data science:
- Machine learning (ML) and artificial intelligence (AI) integration: ML and AI technologies will remain at the forefront of data science. Deep learning, NLP, and computer vision innovations will make it possible to create more sophisticated and clever data-driven applications.
- Automated Machine Learning (AutoML): The creation of AutoML frameworks and tools will streamline the creation and application of machine learning models. These tools speed up the model creation process and automate various steps in the machine learning pipeline, making it easier for non-experts to use.
- Augmented Analytics: To automate data preparation, insight production, and report generation, augmented analytics integrates classical analytics with machine learning and natural language processing. Business users are given the ability to quickly access and understand data, facilitating quicker and better-informed decision-making.
- Internet of Things (IoT) Analytics: As IoT devices proliferate, the amount of data produced by sensors, wearables, and other connected devices will exponentially expand. In order to derive valuable insights from this enormous volume of IoT data, data science will be essential. This will result in increased productivity, predictive maintenance, and individualised experiences.
- Edge Computing and Real-Time Analytics: Edge computing, which places data processing near to the data source, will spread. Real-time analytics will be made possible at the edge by the use of data science techniques, enabling businesses to act quickly based on the most recent data.
- Explainable AI and Ethical Considerations: Interpretability and explainability of AI models will increasingly be stressed as AI becomes more prevalent. For the responsible and reliable deployment of data science solutions, it will be essential to ensure openness and address ethical issues including bias, fairness, and accountability.
- Data Privacy and Security: Data science will need to adapt as data privacy laws change and customers seek more control over their data. For guaranteeing data security while still gaining insights from sensitive data, innovations in privacy-preserving approaches, federated learning, and differential privacy will be crucial.
- Data Science as a Service (DSaaS): As data science skills become more widely available as a service, businesses will be able to deploy advanced analytics and machine learning without having to hire a lot of in-house specialists. Businesses will have simple access to and use of pre-built models, data pipelines, and analytical tools thanks to DSaaS platforms.
- Cross-Disciplinary Collaboration: Data scientists, subject matter experts, and business stakeholders will continue to need to work together. Creating interdisciplinary teams and tearing down silos will improve data interpretation and result in more effective data-driven decision-making.
- continual Learning and Lifelong Education: To remain relevant, professionals will need to embrace continual learning and upskilling given the rapid breakthroughs in data science. The importance of continuing education, online learning, and specialised certifications will rise for data scientists and other professionals looking to enter the area.
Overall, exciting new opportunities, technological developments, and an increasing emphasis on the ethical and responsible use of data will shape the future of data science. Data science will continue to be crucial in defining diverse industries and fostering innovation as businesses increasingly recognise the importance of data-driven insights.
How Ways and Means Technology can help you implement Data Science and Analytics in your business ?
Introducing Ways and Means Technology: Your Partner in Data Science and Analytics Transformation
Are you ready to unlock the full potential of your business through data science and analytics? Look no further than Ways and Means Technology, your trusted partner in driving data-driven success. With our cutting-edge solutions and expertise, we empower businesses like yours to harness the power of data for informed decision-making, improved efficiency, and sustainable growth.
Here’s how Ways and Means Technology can help you implement data science and analytics into your business:
- Customized Data Strategy: We understand that every business is unique, which is why we start by developing a customized data strategy tailored to your specific goals and challenges. Our team of experienced data scientists and consultants will work closely with you to identify key objectives, define metrics, and design a roadmap that aligns with your business needs.
- Comprehensive Data Analytics Solutions: Leverage our comprehensive suite of data analytics solutions to gain actionable insights from your data. From data collection and cleansing to advanced analytics and visualization, we offer end-to-end services that enable you to extract valuable knowledge from your data assets.
- Advanced Machine Learning and AI: Stay ahead of the competition with our advanced machine learning and AI capabilities. Our experts are well-versed in the latest algorithms and techniques, allowing us to build robust predictive models, automate processes, and uncover hidden patterns that drive business growth.
- Scalable Infrastructure and Cloud Solutions: We provide scalable infrastructure and cloud solutions to handle your data processing needs. Whether it’s setting up a cloud-based data warehouse or implementing a distributed computing environment, we ensure your infrastructure can handle growing data volumes and support real-time analytics.
- Data Visualization and Reporting: Make data-driven decisions effortlessly with our intuitive data visualization and reporting tools. Transform complex data into visually appealing dashboards and reports, enabling you to understand trends, identify opportunities, and communicate insights effectively to stakeholders at all levels.
- Data Governance and Compliance: We prioritize data governance and compliance to ensure the security and ethical use of your data. Our team adheres to industry best practices and regulatory standards, helping you mitigate risks, maintain data privacy, and build trust with your customers.
- Training and Enablement: We believe in empowering your team to become data-savvy. Through customized training programs and workshops, we equip your employees with the necessary skills and knowledge to leverage data science and analytics tools effectively, fostering a data-driven culture within your organization.
- Ongoing Support and Optimization: Our commitment doesn’t end with implementation. We provide ongoing support, monitoring, and optimization of your data science initiatives. We continuously analyze and fine-tune models, adapt to evolving business needs, and ensure that your data science capabilities remain at the forefront of innovation.
Don’t let valuable insights hide within your data any longer. Partner with Ways and Means Technology and unleash the power of data science and analytics for transformative business outcomes.
Contact us today to schedule a consultation and take the first step towards a data-driven future with Ways and Means Technology. Together, we’ll navigate the realm of data science and analytics, revolutionizing the way you do business.